This article first appeared on OneStream Software blog page by Tiffany Ma
Introduction
Have you ever wondered what makes a cake delicious? At the core, that deliciousness comes from the combination of ingredients and the process of baking. Now think about the world of machine learning (ML) where the “ingredients” are characterized as “features.” To better understand this notion, let’s delve into our kitchen (or the world of ML) with a simple analogy for FP&A (Financial Planning & Analysis) teams to consider.
Imagine you’re crafting a cake. The recipe (or model in ML) you’re following demands certain ingredients: flour, sugar, eggs and maybe some cocoa for a chocolate spin. Each of these ingredients, with their unique taste and texture, plays a role in determining the final taste, appearance and consistency of the cake.
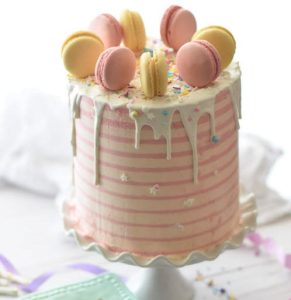
The Cake: The outcome or prediction.
The Recipe: The machine learning model.
The Ingredients: The features.
The absence of sugar might result in a bland cake. In the same way, missing or poorly chosen ML features can weaken a FP&A) model’s predictive accuracy. The right features ensure that won’t happen, and in this second post in our AI for FP&A series, we cover the key ML features that can be your secret ingredient to achieving effective planning and analysis.
Key Ingredients: Features
Features are the building blocks of ML. These individual properties or characteristics are extracted from data and used as input in ML. In the context of time series forecasting, the term “feature” usually refers to attributes derived from time series data that can be used to make future predictions. Well-chosen features can help machine learning models do the following:
- Capture Information: The right information can help identify patterns, trends, seasonality and cyclicality in data
- Incorporate External Knowledge: Time series forecasting often benefits from external information (e.g., events, holidays or other external factors), which features can then integrate to improve forecasting accuracy
- Enhance Interpretability: Some features can help make the model’s decision more interpretable (e.g., a feature that captures the day of the week can easily explain weekly seasonality in sales data)
- Improve Model Accuracy: A well-chosen feature can reduce the complexity of the problem for the model, helping it generalize better and improve accuracy (e.g., using lagged values or rolling averages can help capture temporal dependencies in time series data)
Time series data is often complex and noisy, and machine learning models can sometimes struggle to learn from such data without the right features.
Sensible ML: Taking Baking to the Next Level with Easy-to-Follow Reminders
Let’s be honest: Baking isn’t always easy. Sometimes we might forget an ingredient, miscalculate the proportions or simply not know which ingredients will work best for a particular type of cake. Similarly, in ML, selecting and generating the right features is often a cumbersome, time-consuming process.
But it doesn’t have to be. Keep the following reminders top of mind to take your baking (i.e., ML modeling) to the next level.
Reminder #1: Automate with Sensible ML’s groundbreaking Auto AI capabilities.
Sensible ML understands the challenges associated with features in the ML process and offers auto ML to simplify the process.
Sensible ML is a machine learning solution that automates many of the tasks involved in building and deploying ML models. One of the key features of Sensible ML is its automated feature generation and selection capabilities.
In fact, Sensible ML can automatically generate thousands of features from your data, even if your data is complex or unstructured. For example, assume US housing starts or housing permit activity is a “feature” to help forecast a manufacturer’s door sales. Sensible ML will automatically calculate whether a 1-month, 2-month or longer lead or lag helps improve forecast (door sales) accuracy.
After generating features from your data, Sensible ML can automatically select the most important features for your machine learning model. The benefit? Both the accuracy and efficiency of your model are improved.
Reminder #2: Accelerate insights with the Low-Code/No-Code (LC/NC) Sensible ML Feature Library
Without Sensible ML’s LC/NC Feature Library, Finance teams and data scientists would be forced to identify external data sources on their own, manage each integration and spend time cleaning data. And all that would have to be done before doing all the hard work to gauge whether the contributed external data is useful for forecasting.
Alternatively, Sensible ML accelerates time to value, reduces technical overhead and increases productivity with few, if any, programming skills required. This functionality/feature has saved our customers from needing 2 weeks of a dedicated data scientist’s time.
With Sensible ML’s built-in LC/NC capabilities, Finance teams and analysts can quickly build time-series ML models ready for consumption across the organization – and do it in a way everyone can understand and use.
Sensible ML’s Feature Library thus enables Finance and Operations teams to enrich data using predefined external sources such as the Consumer Price Index, weather or gas prices – without lengthy complicated code only data scientists would understand (see Figure 1). Sensible ML will then do all the “hard work” to identify which of these external sources/variables are relevant to contribute to forecast model performance – and to what extent.
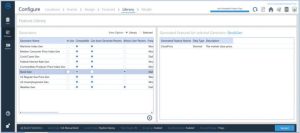
Figure 1: Sensible ML Feature Library
Reminder #3: Use dashboards to visualize forecasts and assess feature impact.
Automatically bringing in features for a more accurate prediction is great. But if there’s no transparency into how the ML model got to the numbers, then what’s the point? Business analysts want to take a forecasted number and explain how they arrived at that prediction, so the organization can develop a strategy going forward. For example, if a sales promotion at a particular time of the year had a large positive impact on sales, this information is valuable for the business.
To that end, Sensible ML comes with several dashboards highlighting feature influence across the portfolio or on individual predictions for each product-location combination (see Figure 2). The Feature Impact dashboard below shows that the “Week of the year” feature has a relative importance of 11.63% to the prediction amount, which is relatively high compared to the rest of the features.

Figure 2: Sensible ML Feature Impact
To take transparency a step further, Sensible ML also shows for any given forecast datapoint what features are driving the forecast higher or lower (see Figure 3).
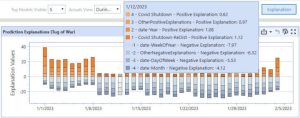
Figure 3: Sensible ML Prediction Explanations (Tug of War)
Forecast accuracy is the de facto outcome of Sensible ML, although deep insights are the much richer benefit our customers are enjoying. Customers can now better understand the specific impacts that external and promotional factors had on product demand. In turn, this deep insight enables more strategic decision-making that results in material impacts to an organization’s financials.
Conclusion
In essence, ML is a lot like baking in that both are a combination of art and science. The right features (or ingredients) can make all the difference. And with Sensible ML’s Auto AI capabilities, the intricate process of building an ML forecast (or baking) is streamlined, ensuring your “cake” is always the talk of the town!
Learn More
To learn more about the end-to-end flow of data and how FP&A interacts with data, stay tuned for additional posts from our Sensible ML for FP&A blog series. You can also download our white paper here.